In recent years, the term "open source" has been thrown around frequently, especially in the context of artificial intelligence (AI). Many projects tout the benefits of open-source methodologies, suggesting an inclusive, collaborative environment that encourages innovation and shared knowledge. However, despite this appealing narrative, not all so-called "open source" AI projects are truly open in nature. It is essential to examine the nuances that lie beneath the surface to understand what "open source" actually means in today’s digital landscape.
The Illusion of Openness
When we think about open source, we often envision a community-driven project where contributions can be made by anyone. However, the reality is more complicated. Many AI projects that label themselves as open source often operate under a model that restricts access in various ways. This could involve selective sharing of code, proprietary licensing terms, or limitations on redistribution, all of which hinder the collaborative spirit that open source ideally promotes.
Open source should mean that anyone can inspect, modify, and enhance the code. However, some AI providers offer only snippets of their source code or release models without adequate documentation, which makes it difficult for developers to contribute meaningfully.
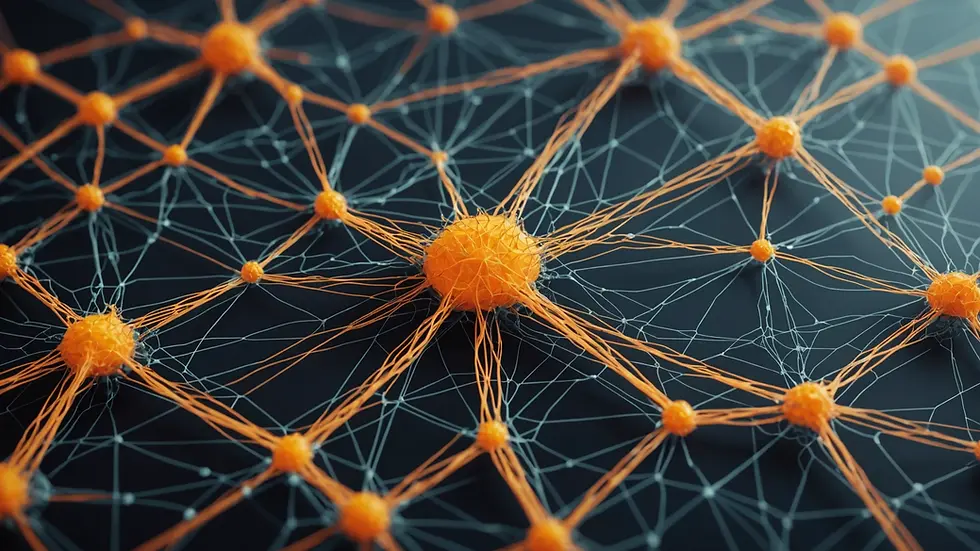
Understanding Access and Control
In the traditional open-source model, development is decentralized; contributors across the globe collaborate freely. However, many so-called open-source AI projects remain tightly controlled by a select few organizations. This consolidation can be contrary to the ethos of transparency and equity central to open-source principles.
Data privacy laws and various compliance standards also come into play. While source code might be open, data access could be tightly controlled, putting a damper on the community's ability to test and enhance the AI models effectively. This limitation can create an environment where only a privileged few can truly gain insights or make impactful changes.
The restrictions surrounding data can lead to the entrenchment of certain models, stifling competition and innovation in the field. The apparent openness can become an illusion when these underlying conditions are considered.
Selective Transparency in AI Models
Transparency in algorithms is pivotal for building trust and accountability. Even when open-source AI models are available, their inner workings may remain obscure. Many developers and organizations struggle to understand the methodologies behind how decisions are made, which is crucial, especially in high-stakes applications like healthcare and finance.
When companies release open-source frameworks but hold back on critical information regarding model training, bias mitigation techniques, and evaluation metrics, it raises ethical and trust issues in the community. Selective transparency can limit an AI practitioner’s ability to assess a model's robustness fully and, consequently, its suitability for deployment in real-world situations.
Moreover, the hype around popular open-source frameworks can often overshadow smaller, community-driven projects that may prioritize transparency and true collaboration. As a result, some truly open-source AI initiatives fade into the background, unable to compete with the marketing might of larger entities.
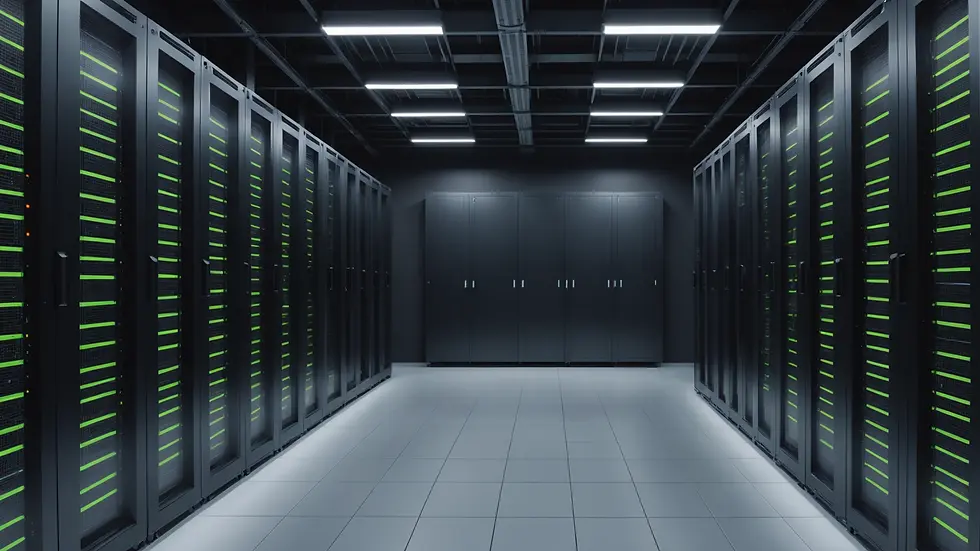
The Challenge of Commercialization
Another layer of complexity surrounding open-source AI lies in the business models of the companies that create these tools. In many instances, businesses pivot to a hybrid model that combines open-source components with proprietary elements. This could manifest as offering an open-source base while providing premium features behind a paywall.
While this approach can financially sustain an organization, it creates ambiguity about what is genuinely open-source. The licenses governing these projects might impose limitations on modifications or commercialization by others, thus impeding the widespread application and innovation that true open-source communities aim to achieve.
Finding a balance between monetization and genuine collaboration remains a challenge. The allure of financial success can conflict with the foundational principles that drive open-source initiatives, leading to disillusionment among community members who believe in a collective, equitable innovation.
Real-World Implications
The ramifications of these challenges extend beyond the development community. Ethical considerations arise when AI models trained on biased data sets make erroneous decisions based on faulty algorithms. When AI isn’t fully open, it exacerbates issues of accountability, as users may not know if or how these biases exist within the system.
Imagine a scenario where a healthcare AI analyzes patient data but is built on a proprietary model with undocumented biases. If something goes wrong, who is responsible? The opacity that sometimes accompanies open-source AI can undermine the very safety nets that the technology aims to provide.
Communities and developers must grapple with these ethical dilemmas and advocate for true openness in AI. Focusing on diverse data gathering, iterative model improvements, and rigorous transparency is essential.
Moving Toward True Openness
To foster a genuinely open source AI landscape, stakeholders across the board must promote the values that define true open source—transparency, inclusiveness, and unrestricted access. Leveraging governance structures, community-driven initiatives, and collaborative platforms will be crucial in realizing these values in practice.
Engaging in discussions around licenses, encouraging diverse data representation, and creating comprehensive documentation are vital steps to reclaiming the spirit of open source. By fostering a culture of cooperation rather than competition, the AI community can leverage its collective strength to build robust, responsible, and transparent systems.
Conclusion
The phrase "open-source AI" may roll off the tongue easily, but behind it lies a complex reality that often obscures the truth. As we navigate this evolving field, it is essential to differentiate between genuine open-source initiatives and those shrouded in selective transparency and hidden controls.
By advocating for true openness and fostering collaborative environments, the community can pave the way for ethical, innovative, and accessible artificial intelligence that truly embodies the essence of open-source philosophies. Together, we can dismantle the barriers and unlock the potential that lies within the true open-source foundation of AI.